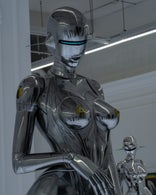
It all began innocuously enough—a regular day in the life of an ordinary livestreamer. This particular stream wasn’t a high-production cooking show or a quirky, scripted internet event. It was just a guy, at home, attempting to make toast. Yet, within hours, this seemingly insignificant act had become a viral sensation, attracting thousands of viewers and generating hundreds of thousands of dollars in ad revenue. The reason? Two AI systems locked into a bidding war for ad placement alongside the livestream.
The two AIs in question were run by competing ad networks, both programmed to optimize ad spend and maximize engagement by outbidding rival systems for premium placements. Initially, both algorithms picked up on some early signs of engagement—perhaps a few viewers stumbled across the stream out of curiosity—and misinterpreted these signals as indicators of high-value content. In response, both AIs began bidding higher and higher prices for the ad spots surrounding the livestream, mistakenly believing that this was the next big viral moment.
As more money poured into the stream, more people tuned in, driven either by curiosity or by sheer confusion over why this simple, mundane event was trending. This, in turn, reinforced the AIs' belief that they were backing a winner. The more viewers joined, the more ad prices skyrocketed, and the cycle continued. What had started as an ordinary livestream was now a full-blown viral event, fueled almost entirely by the whims of two competing algorithms.
How AI Feedback Loops Amplify Meaningless Content
At the heart of this bizarre incident lies a fundamental aspect of how AI-driven advertising works: feedback loops. Feedback loops occur when AI systems learn from past interactions and adjust their behavior accordingly. For instance, if an AI system notices that a particular piece of content is driving higher engagement, it will allocate more resources—such as ad spend—to that content. This reinforcement mechanism helps the system fine-tune its strategies over time, ideally leading to more efficient and effective ad placements.
However, as this incident shows, feedback loops can go wrong. When two or more AI systems are competing in the same space, they can amplify each other’s behavior in unexpected ways. In the case of the toast-making livestream, the two AIs both interpreted the initial engagement as a sign of valuable content, leading them to outbid each other for ad placement. This bidding war drove more traffic to the stream, which in turn reinforced the AIs’ belief that they were backing the right content.
As the feedback loop intensified, the system became more and more detached from the actual value of the content. The algorithms weren’t capable of understanding that the livestream was, in reality, quite boring and irrelevant. They only saw the rising numbers—views, clicks, ad revenue—and concluded that this was where their ad dollars should go.
The Broader Implications of AI-Driven Ad Bidding Wars
While this incident might seem like an isolated fluke, it raises important questions about the role of AI in shaping the digital media landscape. As AI systems become more prevalent in advertising, they are gaining more control over what content gets promoted, how much ad revenue flows to creators, and which pieces of media capture the public’s attention.
One of the key dangers of AI-driven ad systems is their tendency to prioritize engagement metrics over content quality. In the case of the toast livestream, the AI systems didn’t care whether the content was compelling, informative, or entertaining—they only cared about the numbers. As long as the engagement metrics kept climbing, the AIs kept bidding.
This has troubling implications for the future of digital content creation. If AI systems are more concerned with maximizing engagement than with curating quality content, we could see a shift towards more superficial, attention-grabbing media at the expense of deeper, more meaningful material. In other words, content creators may feel pressure to produce whatever drives clicks, rather than what contributes to a rich and diverse media ecosystem.
The Human Cost of AI-Driven Advertising
The effects of AI-driven advertising are not limited to quirky viral moments like the toast-making livestream. In fact, the human cost of these systems can be significant, particularly for small and independent content creators who rely on digital ad revenue to sustain their work. As AI systems compete for ad placements, they can drive up costs in ways that distort the market and make it harder for smaller creators to compete.
For example, if an AI system locks onto a particular piece of content—like the toast livestream—and begins bidding aggressively for ad space, it can cause a ripple effect that raises ad prices across the board. This means that smaller creators, who may not have the same level of engagement, could see their ad revenue decline as larger, AI-boosted events soak up the available ad spend.
This creates a paradox: while AI systems are theoretically designed to make advertising more efficient, they can also create inefficiencies by distorting the market and driving up costs for smaller players. In the long run, this could lead to a less diverse media landscape, as independent creators struggle to keep up with the algorithmic arms race.
The Future of AI in Advertising: Can We Fix the System?
So, what can be done to prevent incidents like the toast-making livestream from becoming the norm? One potential solution is to introduce more human oversight into the AI-driven advertising process. While AI systems are excellent at processing vast amounts of data and making real-time decisions, they often lack the contextual understanding needed to make nuanced judgments about content quality.
By incorporating human input into the process—whether through manual ad curation or more sophisticated AI systems that can assess content on a deeper level—advertisers can help ensure that their ad spend is being directed towards meaningful, high-quality content, rather than superficial viral sensations.
Another potential solution is to design AI systems with built-in safeguards that prevent them from getting trapped in feedback loops. For example, AI systems could be programmed to recognize when they are bidding too aggressively on a particular piece of content and adjust their behavior accordingly. This would help to prevent runaway bidding wars and ensure that ad spend is distributed more fairly across the digital media landscape.
The story of the toast-making livestream is both hilarious and unsettling, offering a glimpse into a future where AI systems control not just the flow of digital ad dollars, but the very nature of the content we consume. As AI continues to shape the digital media landscape, incidents like this one will likely become more common—highlighting the need for greater oversight, more sophisticated algorithms, and a renewed focus on content quality.
Ultimately, the future of AI-driven advertising will depend on our ability to balance efficiency with fairness, and engagement with value. If we can get that balance right, we may be able to harness the power of AI to create a more diverse, engaging, and sustainable digital media ecosystem. If not, we may find ourselves living in a world where making toast becomes the next great internet sensation—for all the wrong reasons.
Add comment
Comments